Welcome to the Treehouse Community
Want to collaborate on code errors? Have bugs you need feedback on? Looking for an extra set of eyes on your latest project? Get support with fellow developers, designers, and programmers of all backgrounds and skill levels here with the Treehouse Community! While you're at it, check out some resources Treehouse students have shared here.
Looking to learn something new?
Treehouse offers a seven day free trial for new students. Get access to thousands of hours of content and join thousands of Treehouse students and alumni in the community today.
Start your free trial
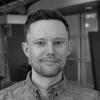
Cody Stephenson
8,361 PointsAre these valid solutions for the individual practice tasks?
OCQ130 has valid values of (1-7, 77, 99). I wanted to included the 77 and 99 so I couldn't use a > [highest valid code]
clause like in the example, so I used.
ind = ocq['OCQ130'].between(7, 77, inclusive=False)
ocq.loc[ind, 'OCQ130'] = np.nan
and for OCQ150 the valid values are (1, 2, 3, 4, 7, 9) but there were some 8's in the unique values so <> wouldn't work, and neither would df.between() so I went with
ind = ocq['OCQ150'].isin([1, 2, 3, 4, 7, 9])
ocq.loc[~ind, 'OCQ150'] = np.nan
These seemed reasonable and so did the subsequent outputs and checks on the data, I just wanted to see if there might be any hidden gotchas I missed.