Heads up! To view this whole video, sign in with your Courses account or enroll in your free 7-day trial. Sign In Enroll
Preview
Video Player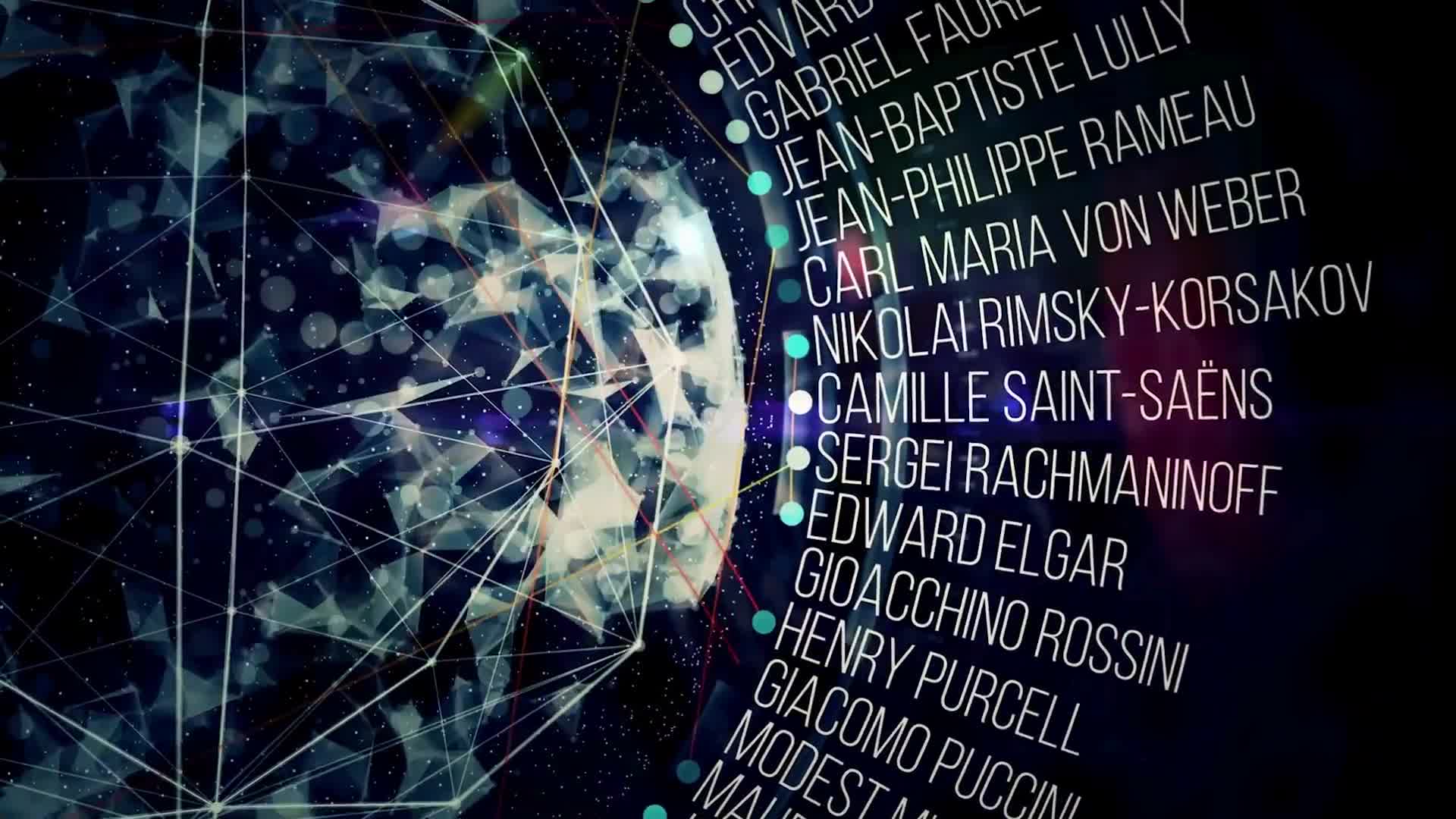
00:00
00:00
00:00
- 2x 2x
- 1.75x 1.75x
- 1.5x 1.5x
- 1.25x 1.25x
- 1.1x 1.1x
- 1x 1x
- 0.75x 0.75x
- 0.5x 0.5x
Navigate the ethical maze of NLP! From data privacy to bias, learn the do's and don'ts in the world of NLP.
Continue Your Learning
Related Discussions
Have questions about this video? Start a discussion with the community and Treehouse staff.
Sign upRelated Discussions
Have questions about this video? Start a discussion with the community and Treehouse staff.
Sign up
The rapid advancement of natural
language processing technologies has
0:00
brought unprecedented
convenience to our lives.
0:03
However, with great power
comes great responsibility.
0:06
As we continue to develop and
deploy NLP systems,
0:10
it's crucial to address the ethical
challenges that accompany this technology.
0:12
This encompasses ensuring that our
systems are fair, accountable and
0:17
transparent, while safeguarding
privacy and security.
0:21
Imagine an algorithm, a set of rules
destined to parse through the nuances of
0:25
human language,
trained on a skewed data set that
0:29
doesn't fully represent the kaleidoscope
of human speech and expression.
0:31
It could be a translation system that
inadvertently perpetuates gender
0:36
stereotypes, favoring masculine pronouns
simply because it learned language from
0:39
a world where professional roles were
portrayed as predominantly male.
0:44
Or consider a machine that,
like an ill-informed traveler,
0:48
stumbles over the colloquialisms and
dialects of a culture,
0:52
its errors a mirror of racial or cultural
biases baked into its digital DNA.
0:55
The repercussions are not
just theoretical.
1:00
Bias in NLP can creep in through the data
we feed our models, the way algorithms
1:02
are designed, or the specific
applications they are put to use in.
1:07
For example, if an NLP system is trained
on text data that under-represents certain
1:11
dialects or demographic groups,
it may perform poorly for those users, or
1:16
worse reinforce harmful stereotypes.
1:20
These biases may manifest in systems that
filter resumes with a bias towards certain
1:23
names and in chatbots that fail to serve
a global audience because they only
1:28
understand a subset of
linguistic subtleties.
1:32
Such biases left unchecked not only
perpetuate existing prejudices but
1:36
also cast a shadow on the credibility and
utility of NLP systems.
1:40
Confronting these biases requires
a vigilant eye and a diverse toolkit,
1:45
regular system audits to detect prejudice,
debiasing techniques to cleanse data, and
1:48
a commitment to collecting as varied and
inclusive a dataset as possible.
1:53
It's about continually refining the lens
through which machines interpret
1:58
our words.
2:01
The ethical narrative of NLP
also speaks to fairness.
2:03
That AI should deliver justice
through unbiased performance.
2:06
It's about holding
creators accountable for
2:10
the digital words put
forth by their creations.
2:12
Transparency becomes the window through
which we can observe the inner workings of
2:15
these intelligent systems, understanding
how they arrive at conclusions and
2:20
how they've been taught to
interpret the human lexicon.
2:24
In this narrative, the plot thickens
with the consideration of privacy.
2:28
Text data, rich with personal information,
can become a tool for
2:33
invasion if not handled with care.
2:36
Pictures secure vaults of encrypted data,
practices of anonymization, and
2:39
the silent guardianship of
regulations like the GDPR,
2:43
all characters in the story of
protecting individual privacy.
2:46
As we look towards the horizon,
the future of NLP is both promising and
2:50
fraught with challenges.
2:54
Neural networks grow more intricate,
their ability to decipher and
2:56
replicate human language more nuanced.
2:59
Techniques on the cusp of development,
such as few-shot learning,
3:01
beckon with the promise of machines
that can learn more with less,
3:05
understand better with fewer examples.
3:08
Yet for all the technical prowess,
these systems may still falter at the deep
3:11
understanding required to navigate
the complexities of human speech and
3:15
the ethical landscapes that accompany it.
3:19
The development of NLP shows humanity's
creativity and complexity, while providing
3:21
many benefits NLP also raises serious
ethical questions about bias, fairness,
3:26
accountability and transparency
that require proactive solutions.
3:30
Advancing NLP ethically demands
commitments to diverse data,
3:35
transparent methods and privacy safeguards
to empower rather than exclude.
3:38
Sustaining public trust and integrity
requires balancing innovation with ethical
3:43
principles so that NLP represents
humanity's best, not worst, instincts.
3:48
And that wraps up our journey
through the ethical maze of NLP.
3:52
Remember, as we continue to harness
the incredible powers of NLP,
3:57
we must also stay vigilant
about using it responsibly.
4:01
I hope you're as excited and
optimistic as me about the future of NLP.
4:04
Thank you for joining us on this
enlightening ride and stay tuned for
4:09
more insightful discussions on AI.
4:12
See you next time.
4:14
You need to sign up for Treehouse in order to download course files.
Sign upYou need to sign up for Treehouse in order to set up Workspace
Sign up